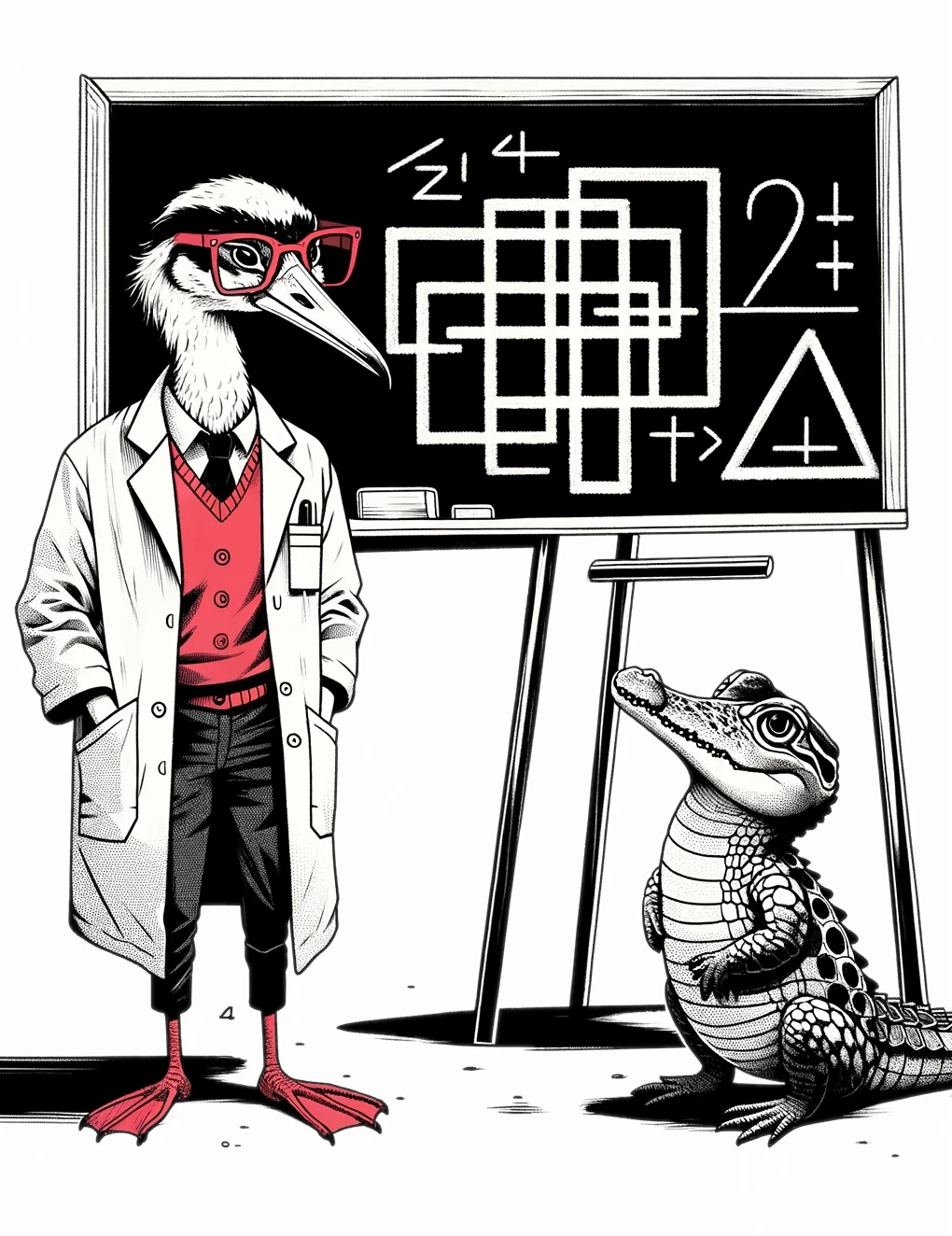
The Egyptian Plover: Rhymes with "over". An African waterbird that maintains a (dubious) symbiotic relationship with crocodies, feeding on decaying meat lodged between their teeth.
Find, explain, and fix bad data & the processes behind them.
From detecting data errors and anomalies to diagnosing process inefficiencies, Plover continuously monitors your business operations via your data systems to automatically identify issues, and improve and maintain the health of your business.
Data aren't messy, processes are.
So-called "messy data" are evidence of messy processes. Plover automatically identifies and explains erroneous and anomalous data to help you find opportunities for organizational effeciency gains.
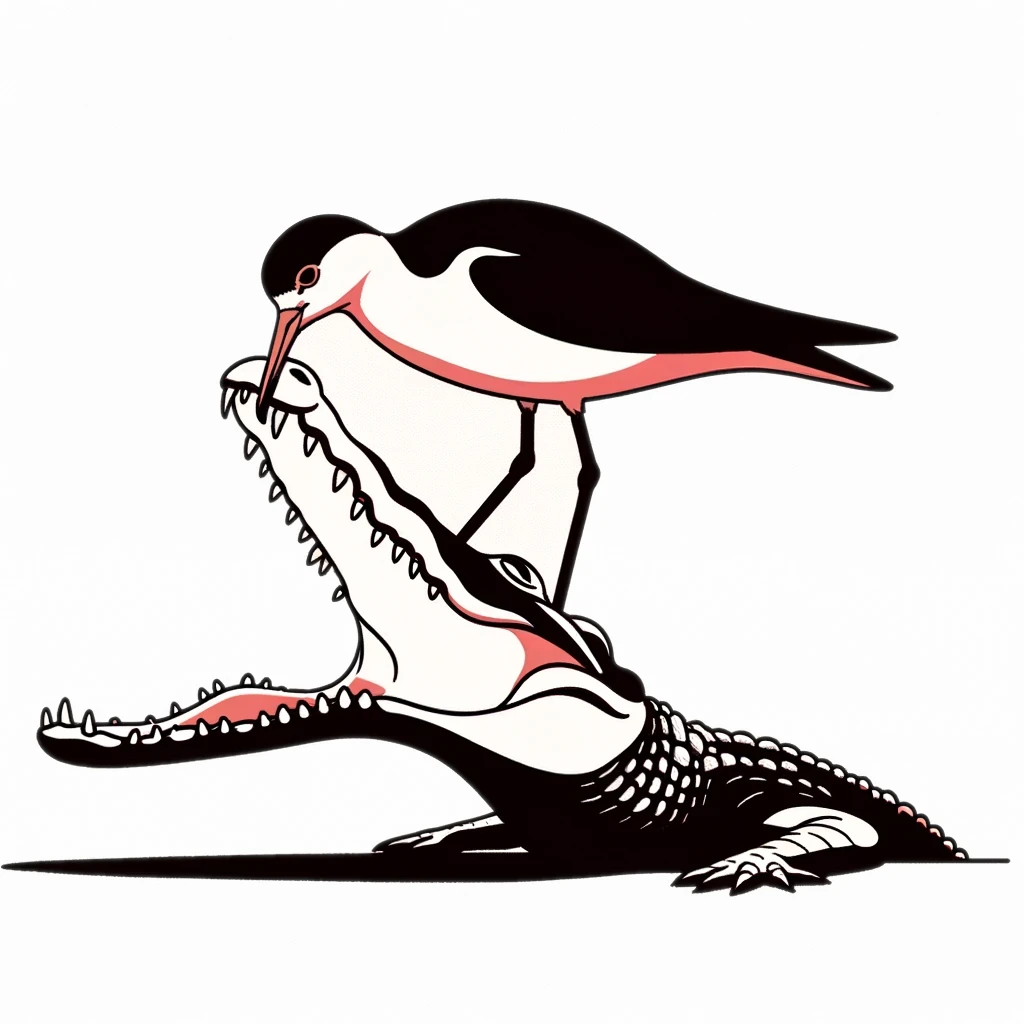
Automatically identify erroneous values
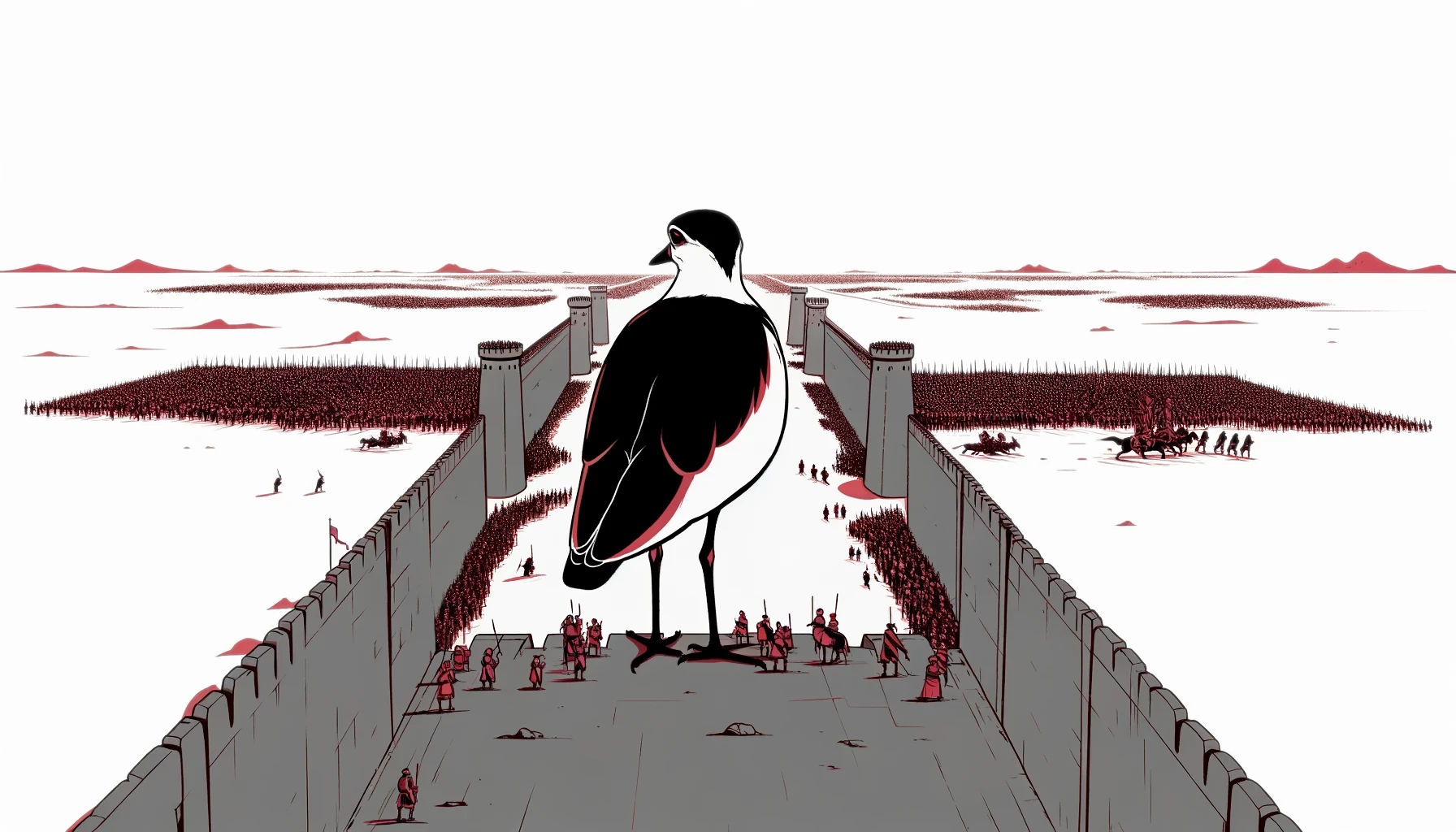
Protect your dataset from future erroneous values
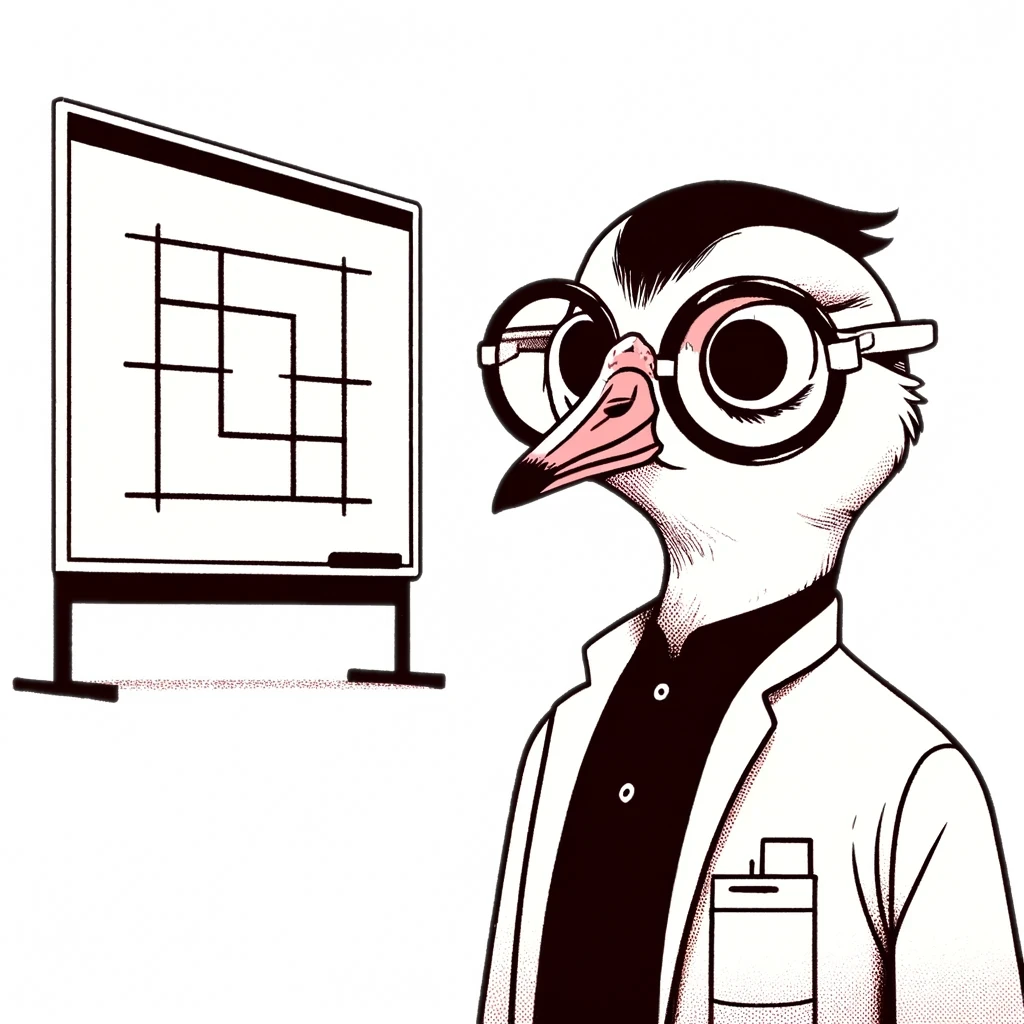
Help diagnose the cause of errors
From deployment to action in a few lines of code
1. Plover builds a model of your data
from plover.source import ImmutableSqlSource
from plover.store import AwsS3Store
from plover.backend import AwsBackend
from plover.engine import DatabaseEngine
conn = connect_to_sql_database()
db_engine = (
DatabaseEngine(
source=ImmutableSqlSource(conn, "SATELLITES_WH"),
store=AwsS3Store("myorg-plover", "SATELLITES_WH"),
backend=AwsBackend("myorg-plover"),
)
.fit()
.persist()
.metalearn()
.persist()
)
Holistic modeling and metalearning
Plover builds a holistic model of the world as defined by your data, then learns a metamodel to further refine its understanding of the information in your data.
2. Identify Likely Errors
top_5_errors = (
db_engine
.metrics
.errorness
.sort(by=['Confusion'], descending=True)
.head(5)
)
Row | Column | Confusion | Observed | Predicted |
---|---|---|---|---|
Intelsat 903 | Eccentricity | 10.514456 | 0.793069999999… | 0.000335826120… |
Intelsat 902 | Inclination_ra… | 10.036763 | 25.06467339 | 0.002274957131… |
Intelsat 903 | Apogee_km | 7.853968 | 358802 | 35792.92656399… |
DSP 20 (USA 14… | Period_minutes… | 6.695287 | 142.08 | 1436.095724064… |
SDS III-6 (Sat… | Source_Used_fo… | 4.93515 | JM/5_11 | ZARYA |
Inconsistency is key
Plover finds errors by identifying data that are inconsistent with its model of your data, and shows you the observed value and its predicted value, which you may use to overwrite erroneous values.
3. Find similar errors
errs_like = db_engine.errors_like(
"DSP 20 (USA 149) (Defense Support Program)",
"Period_minutes"
)
row | rowsim | Observed | Predicted |
---|---|---|---|
SDS III-7 (Sat… | 0.988281 | 23.94 | 1436.088006 |
SDS III-6 (Sat… | 0.964844 | 14.36 | 1436.113453 |
Advanced Orion… | 0.9453125 | 23.94 | 1436.105354 |
Metareasoning
After identifying an error, Plover can identify similar errors by finding data that have similar information profiles.
4. Identify errors in incoming data
err = db_engine.detect(new_satellite_record)
err[0]
Row | Column | Incon Quantile | Observed | Predicted |
---|---|---|---|---|
Satmex 8 | Users | 0.99998 | Commecial | Commercial |
Protect Prod
Quantify how inconsistent data are before they make it into your production data systems
Machine learning + human learning + engineering
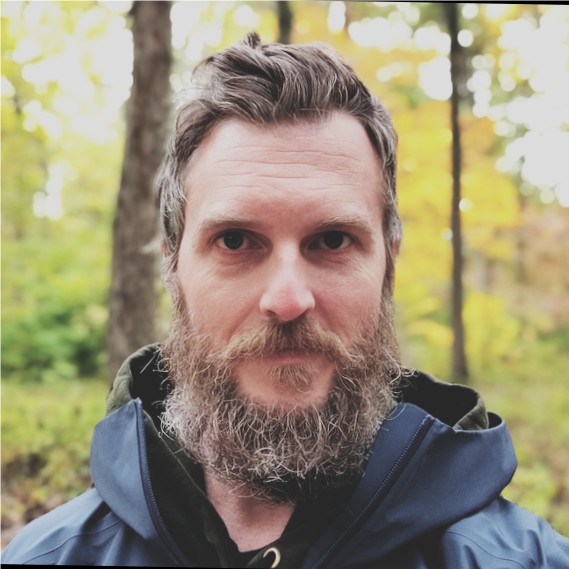
Baxter Eaves
CEO
Baxter is a US Navy veteran and holds a PhD in Experimental Psychology from the University of Louisville where he developed computational models of human trust and social learning. He has led a number of DARPA projects and brings 13 years of experience deploying human-inspired AI tech in high-risk industries.
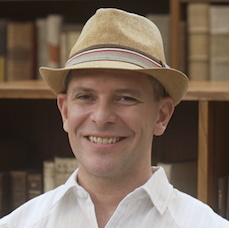
Patrick Shafto
Scientist at large
Patrick is a program manager at DARPA under the Information Innovation office (I20) and professor of Data Sciences at Rutgers University - Newark. He has led a number of projects for agencies including DARPA, DOD, and NSF, and his publications have appeared in top journals of machine and human learning.
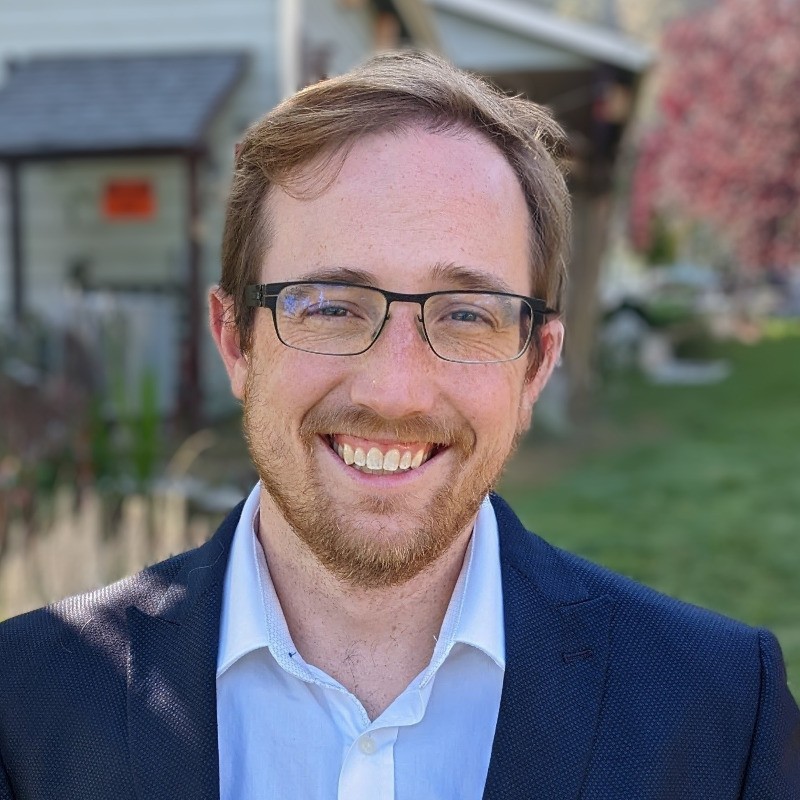
Michael Schmidt
Principal ML Engineer
Michael has 14 years of research and engineering experience. He has built production models for healthcare, agronomy, finance, and law; and has conducted research in the areas of high-energy physics, differential geometry, plasma physics, and high-performance computing.
Frequently asked questions
Is Plover another wrapper around Chat GPT, Claude, llama, etc?
No. Plover is proprietary probabilitstic machine learning model designed for semi structured relational data. Plover is designed to be explainable and to properly quantify uncertainty.
What data is Plover trained on?
Your data and
Is Plover secure?
Plover is installed on your infrastructure whether that be in the cloud or in an air gapped electromagnetically secure environment. Plover is exactly as secure as your systems — no more, no less.
Does Plover transmit my data externally?
No.
Is Plover expensive to train? Does it require special hardware like GPUs?
No. You can train and query a simple Plover model in a couple of minutes on an old iPhone. Don't believe us? Hop over to the demo on your smartphone, download one of the provided datasets, put your smartphone in airplane mode, and test it out.
Get in touch
Provide your information here and we will reach out to you promptly.